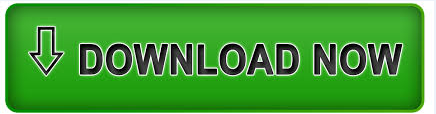
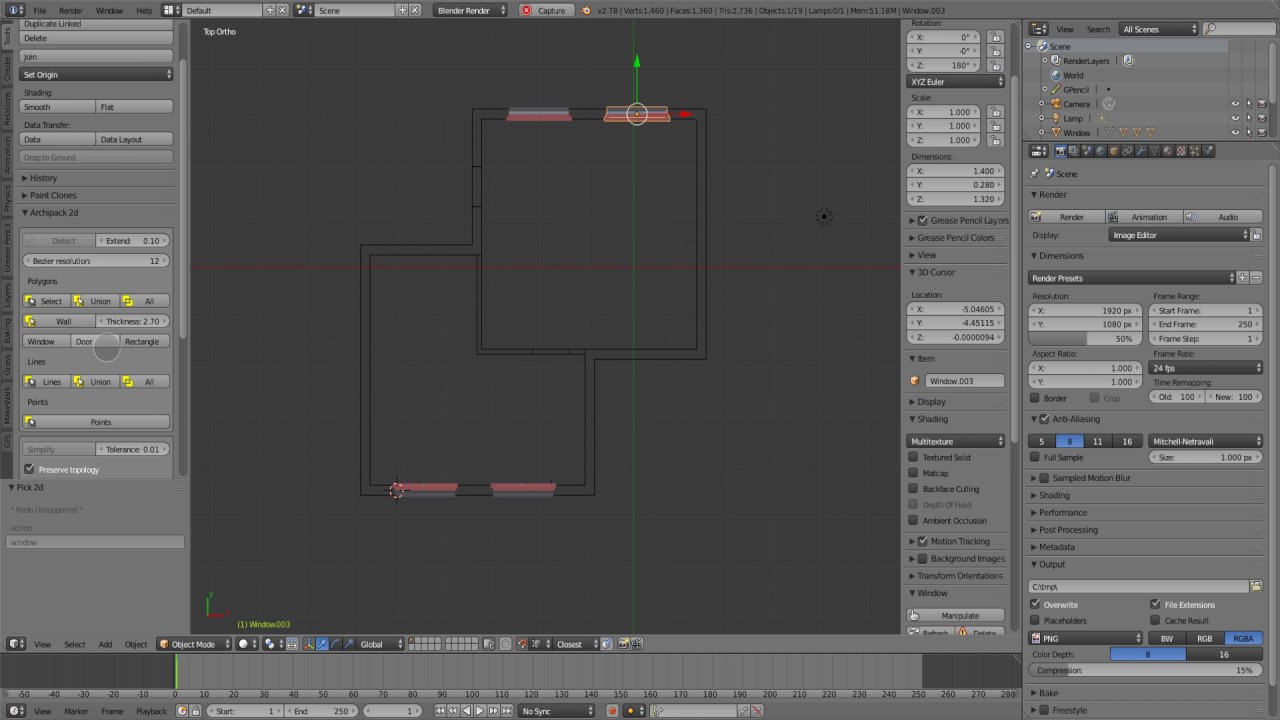
The 2nd button is for selecting an image from a history list. The first button is used for browsing for an image. There are 2 buttons, a text box, and a final button. Image selection is controlled on the row labeled. In v2.43 the equivalent button is the button. When you turn the button on again, your previous settings are back. Turning the button off will not clear the settings it just hides the image.
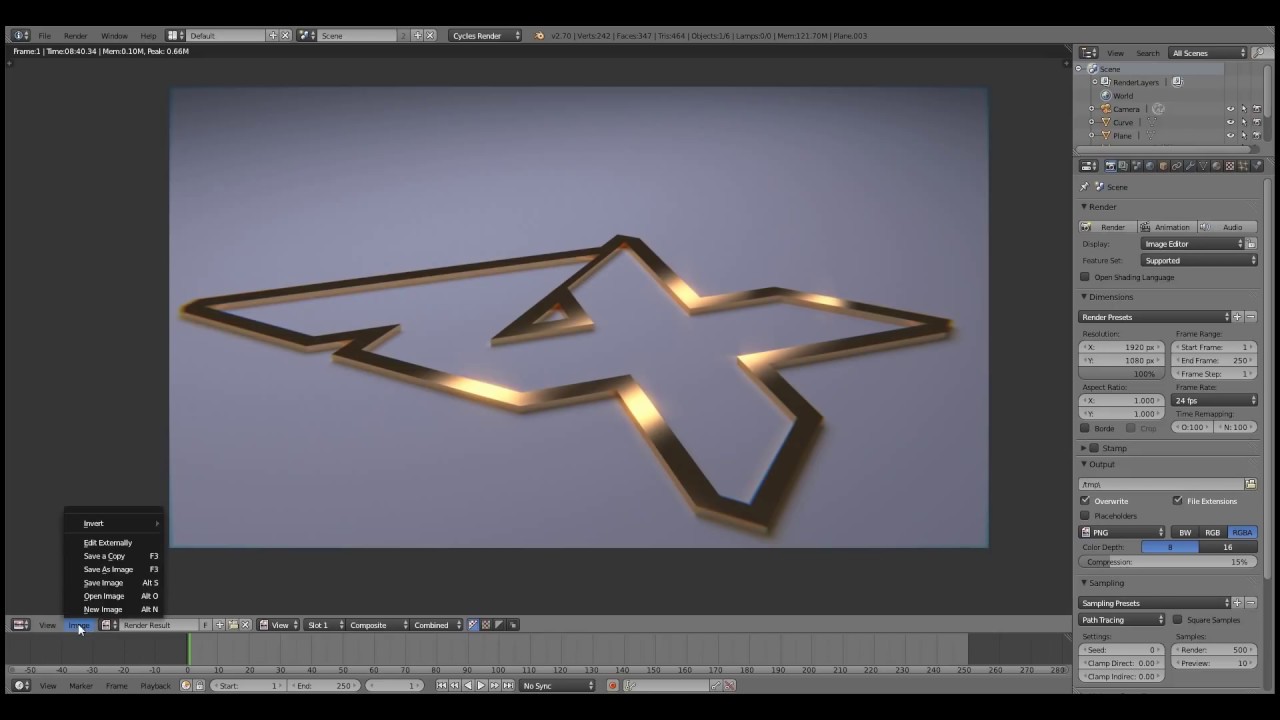
The is a toggle button that turns display of the image on or off. (The image will not be rendered as it is not part of your scene.)ĭetails of the background image panel If perspective view is enabled, toggle to orthogonal view by pressing NUM5. Noob note: The image is only displayed in orthogonal view. Using the navigation techniques from the previous tutorials, find your 2D jpeg image on your computer, click the file in the list once then click the Select Image button at the top right of the screen. You are now presented with a file selection screen. One of them says image: and has a small button with a picture of a folder on it click this button. At the bottom of the 3D viewport on the left, there are some menus, click View -> Background ImageĪ small window will appear containing just one button marked use background image click this button. If you haven't already done so, open blender and select one of the orthogonal view angles by pressing NUM7 (top), NUM3 (side), or NUM1. Our approach requires no 3D training data and no modifications to the image diffusion model, demonstrating the effectiveness of pretrained image diffusion models as priors.Load the background image into blender Basics of the background image panel The resulting 3D model of the given text can be viewed from any angle, relit by arbitrary illumination, or composited into any 3D environment. Using this loss in a DeepDream-like procedure, we optimize a randomly-initialized 3D model (a Neural Radiance Field, or NeRF) via gradient descent such that its 2D renderings from random angles achieve a low loss. We introduce a loss based on probability density distillation that enables the use of a 2D diffusion model as a prior for optimization of a parametric image generator. In this work, we circumvent these limitations by using a pretrained 2D text-to-image diffusion model to perform text-to-3D synthesis. Adapting this approach to 3D synthesis would require large-scale datasets of labeled 3D assets and efficient architectures for denoising 3D data, neither of which currently exist. Recent breakthroughs in text-to-image synthesis have been driven by diffusion models trained on billions of image-text pairs.
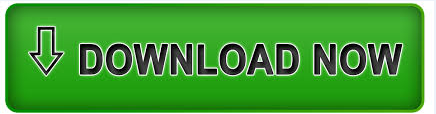